Pre-Conference Training
15 November 2021
2.00 pm to 6.00 pm CET – Online
An Introduction to Causal Inference in Experimental and Observational Settings – Theory and practice
Introduction
Most scientific questions are causal in nature. It is therefore necessary to introduce a formal causal language to help define causal effects and spell out the assumptions required to infer such effects from experimental and observational data.
Programme
The potential outcome approach to causal inference will be introduced and statistical methods for inferring causal effects from randomized experiments or observational studies will be presented. Examples and practical sessions will be based on case studies in biostatistics, epidemiology, and public health.
- Introduction to causal inference from the potential outcome perspective.
- Design and analysis of randomized experiments: Fisher’s exact p-values, estimators of average causal effects, regression, imputation-based approaches.
- Practical session 1: Analyzing an RCT on the effect of statins on cholesterol
- Design and analysis of observational studies under confoundedness: the role of the propensity score; matching, weighting, regression estimators.
- Practical session 2: Analyzing an observational study on the effect of statins
- Beyond RCTs. Intercurrent events: challenges and opportunities. Presentation of a case study with discussion.
Lecture notes, slides, data, articles and other reading material will be distributed before the course.
Practical sessions will be in R but no a priori knowledge of R is required.
Participant experience
Statistical inference, multivariate analysis.
Who should attend?
The course is addressed to Statisticians, health professionals with statistical background, master and PhD students.
Teaching methods
Lectures with some practical sessions/examples.
Lecturer
Fabrizia Mealli – Professor of Statistics, Director of the Florence Center for Data Science at University of Florence
Fabrizia Mealli is Professor of Statistics. Her research focuses on causal inference, program evaluation, estimation techniques, simulation methods, missing data, and Bayesian inference, with applications to the social and biomedical sciences. She held visiting positions at Harvard University, UCLA, LISER Luxembourg. She serves as coordinator of the Statistics track for the PhD program in Mathematics, Computer Science, Statistics of the University of Florence, and sits the Steering Committee of the European Causal Inference Meeting. She is Elected Fellow of the American Statistical Association, and currently an associate editor of “The Annals of Applied Statistics” and “Observational Studies”.
At the end of the training, you will be able to …
… develop expertise to assess the credibility of causal claims and the ability to apply the relevant statistical methods for causal analyses.
Main reference:
Imbens G., Rubin D.B. (2015) Causal Inference for the Statistics, Social and Biomedical Sciences: An Introduction, Cambridge University Press
Register

Printable Version
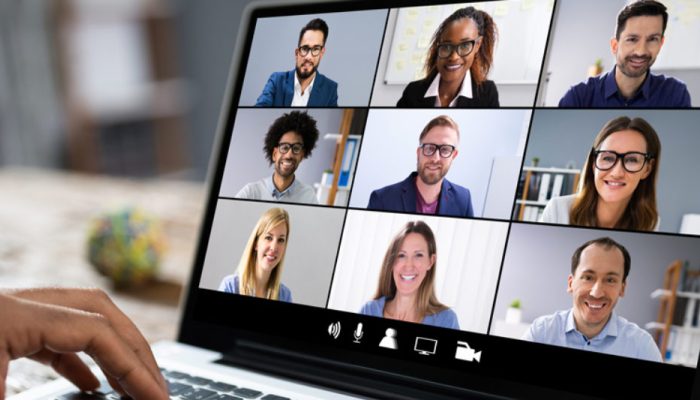